Make what matters
Nuance tackles real‑world challenges to produce transformative, mission‑critical outcomes.
We specialize in conversational AI and ambient intelligence
Automatic Speech Recognition (ASR)
Nuance has played a foundational role in the evolution of speech recognition. Our ASR research team uses deep learning to map audio to text with high fidelity, even in challenging acoustic environments and tight resource constraints, leveraging application knowledge to ensure optimal performance.
Natural Language Processing (NLP)
As pioneers in NLP, we’ve been talking to machines since long before smart phones existed, engineering AI that goes beyond recognizing words to understanding their meaning. Our NLP research uses deep learning to optimize our understanding of typed or spoken user input in the context of an overall dialogue.
Biometrics
Our market‑leading, award‑winning biometrics solutions inform Nuance’s services and products for security and fraud protection. Leveraging parallel deep learning stacks to efficiently and precisely identify or verify speakers and authors based on audio and linguistic content, these AI research streams enable natural, secure interactions.
AI research in action
Our solutions are designed to empower excellence, meet specific needs and requirements, and address the biggest challenges our customers face every day.
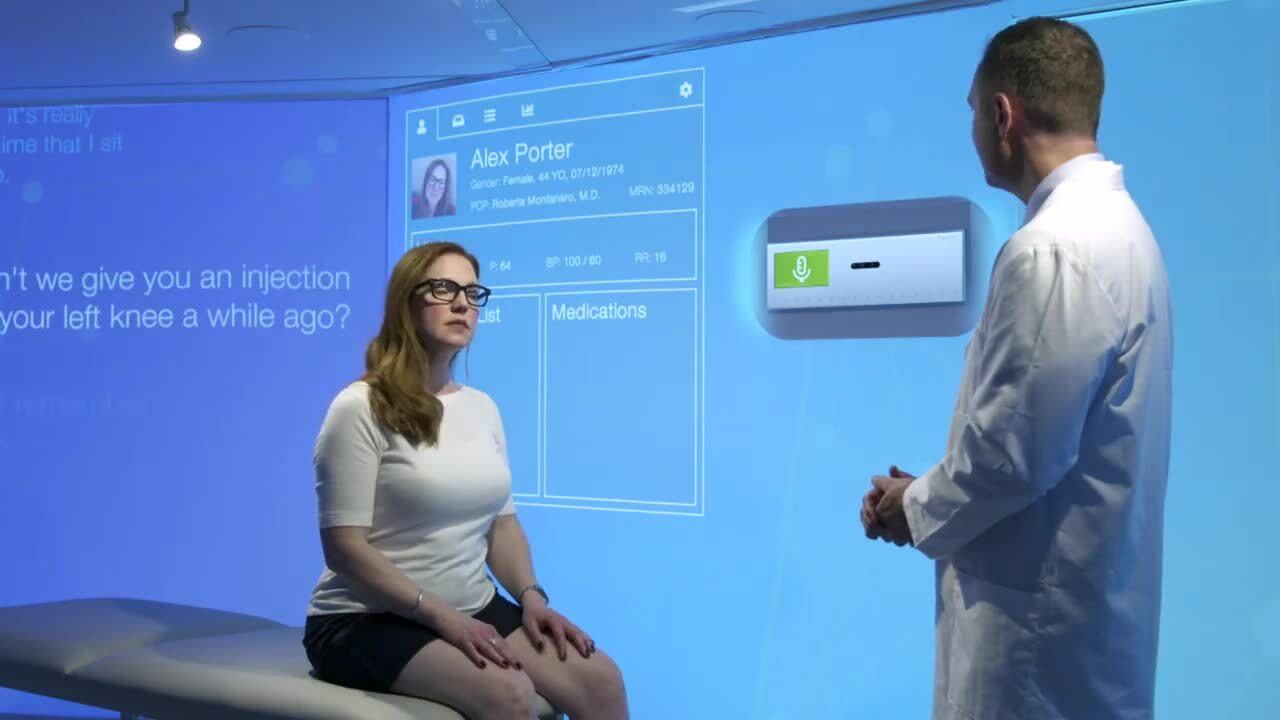
Nuance DAX™ (Play a video)
Powered by cutting‑edge Nuance artificial intelligence, Dragon® Ambient eXperience™ (DAX) provides a seamless ambient experience, unburdening clinicians so they can focus on patient care. Multi‑channel signal processing, far‑field ASR, speaker diarization, and auto‑punctuation create a readable transcript of the patient encounter and abstractive summarization technology is used to produce a properly formatted and structured medical report.
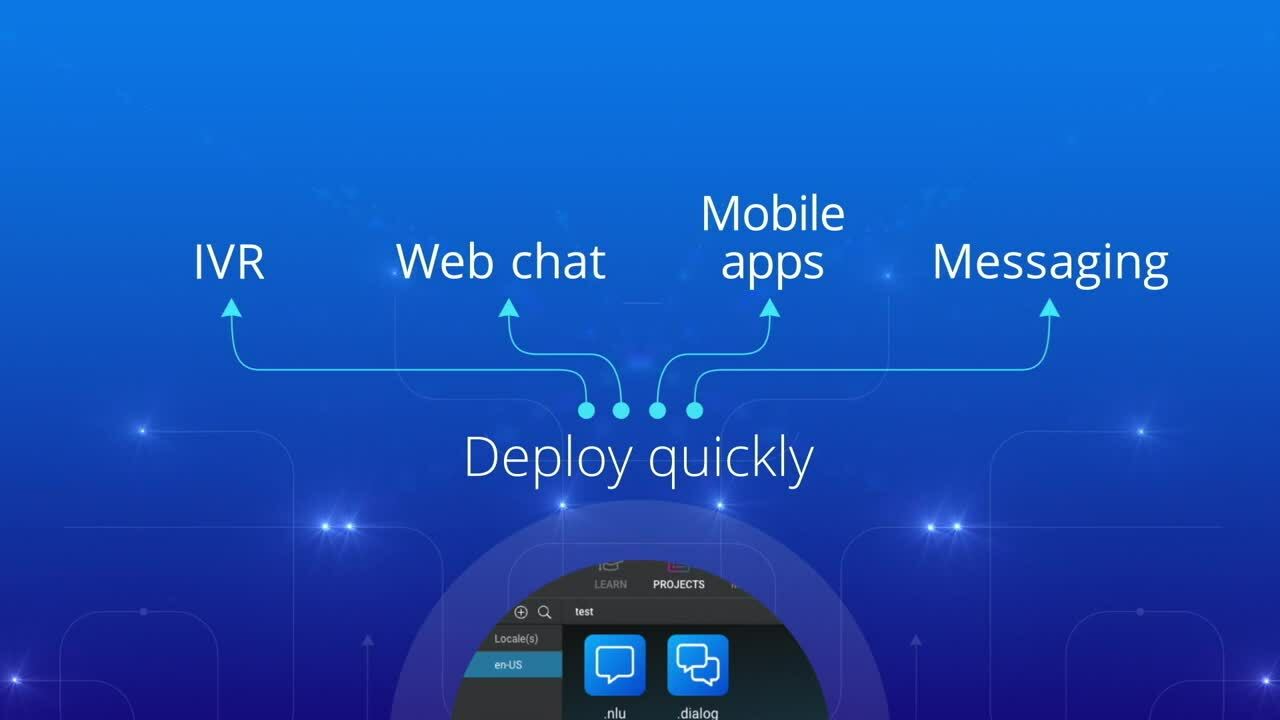
Nuance Mix™ (Play a video)
The Mix™ platform puts the power of our artificial intelligence technology in our customers' hands, creating a custom conversational engagement by adapting speech recognition and contextual language understanding models to each application's needs. We use transfer learning and model adaptation to leverage our vast in‑domain data holdings, minimizing application annotation requirements and improving performance over time.

Nuance Gatekeeper™ (Play a video)
Gatekeeper™ leverages proprietary speaker and author verification and next‑level fraud detection technology to enable frictionless secure communication. To keep ahead of constantly changing fraud techniques, we invest in research to detect audio spoofing via synthesized or morphed speech or playback attacks, evolving our technology to verify that the reported device, channel, and age on file is consistent with observed acoustics.
More data. Smarter AI. Better outcomes.
Our vertical solutions are tailored to industry‑specific needs and protected by world‑class security. Our artificial intelligence evolves to meet changing objectives by drawing on massive streams of data that help us continually learn, improve, and resolve emerging problems.
patient stories captured and communicated annually
customer interactions via digital and voice channels per year
biometric authentications per year

We believe customer data is sacred, so we build our cutting‑edge AI technology responsibly—with data privacy and protection in mind.
Joe Petro
Executive Vice President and Chief Technology Officer
Learn more about our trust principles
Commercial and academic partnerships
Thinking bigger together
We partner with global academic and commercial thought leaders to advance the state of the art in conversational AI.





Engineering excellence
Mapping tomorrow’s technology
Conversational AI isn’t just about building technology, it’s about creative problem‑solving and how you design, develop, and optimize AI to create the best user experience.
Nirvana T.
Director, Software Engineering
Core Technology R&D
Thought leadership
Recent AI publications

Prediction Network Architecture in RNN-T for ASR(Open a new window)
Nuance What's Next blog article by Dario Albesano

Training automatic speech recognition models with de-identified data(Open a new window)
Nuance What's Next blog article by Martin Flechl

Improved far field speech recognition with microphone arrays(Open a new window)
Nuance What's Next blog article by Dushyant Sharma

Combining the advantages of close-talk and far-talk speech recognition(Open a new window)
Nuance What's Next blog article by Felix Weninger